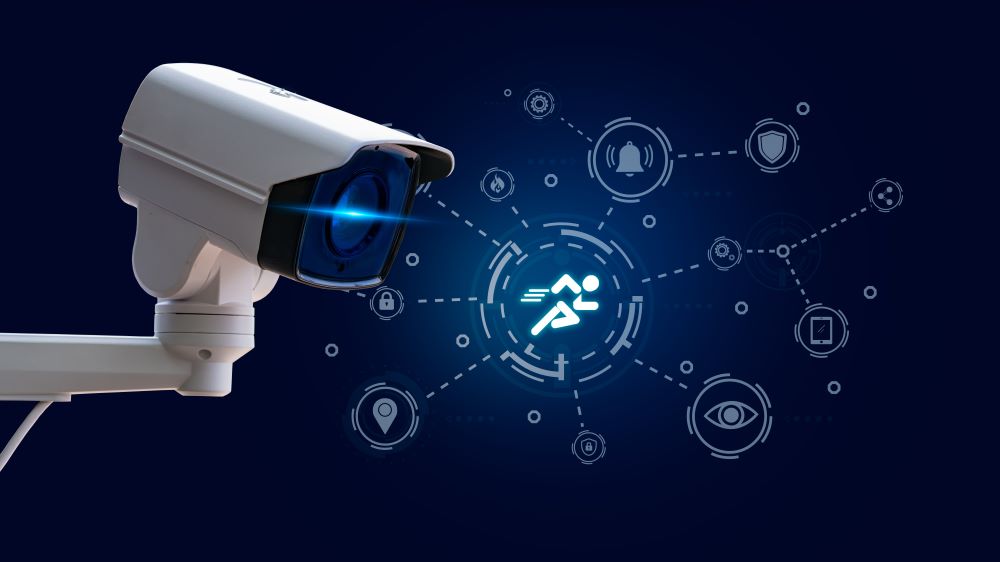
関連するソリューション
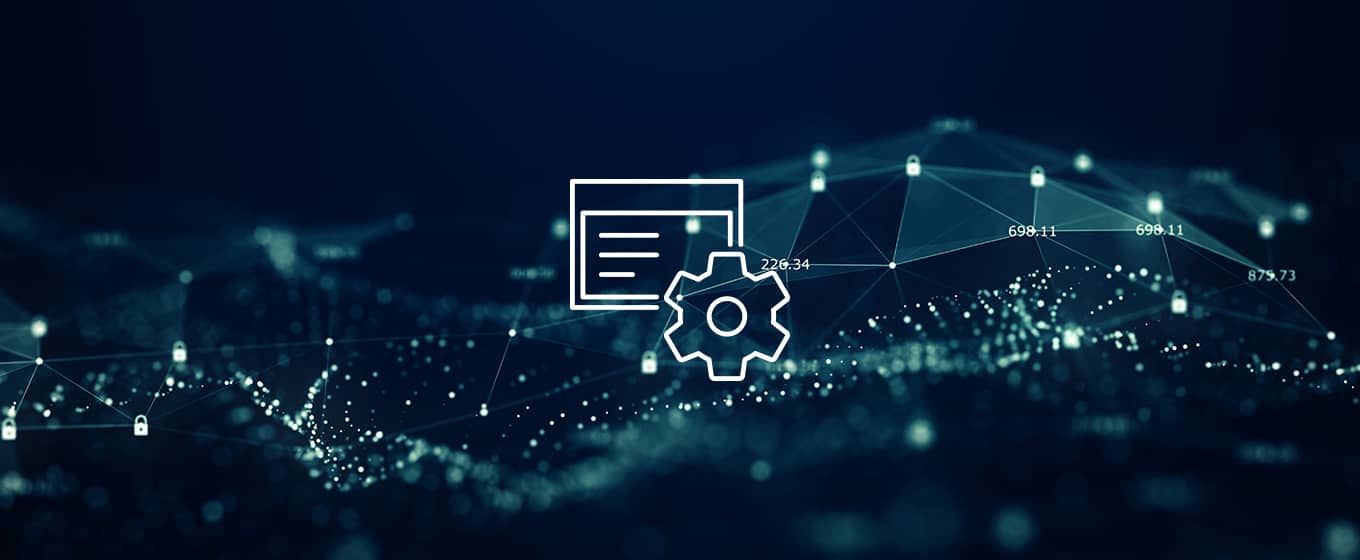
業務改革
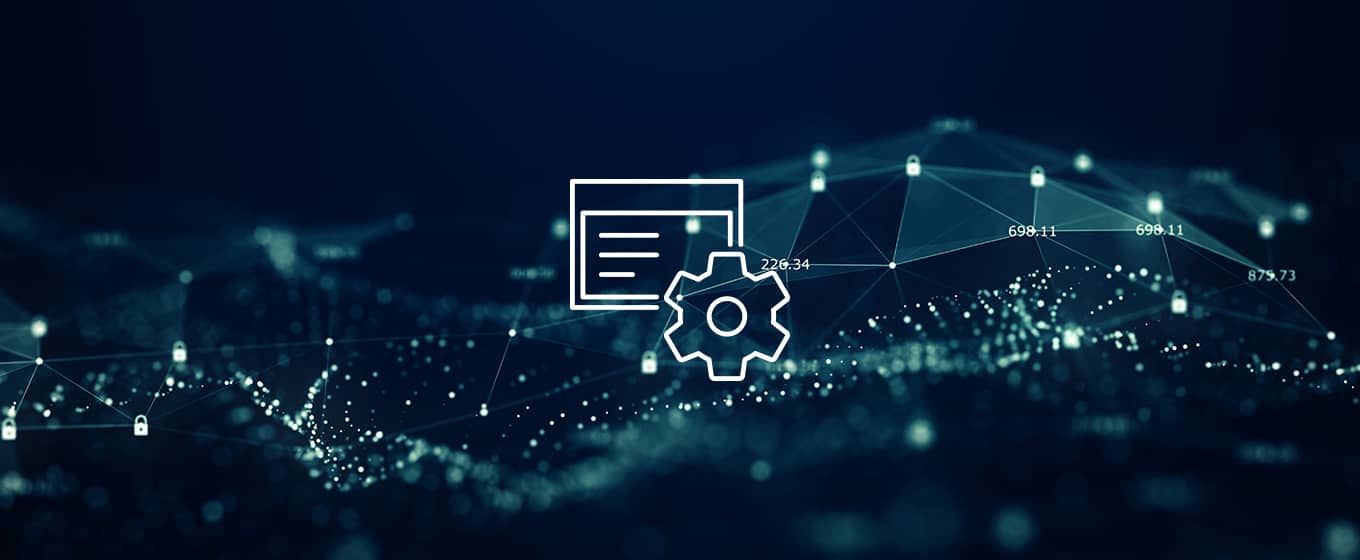
AI
テクニカルスペシャリスト
松尾 大輔 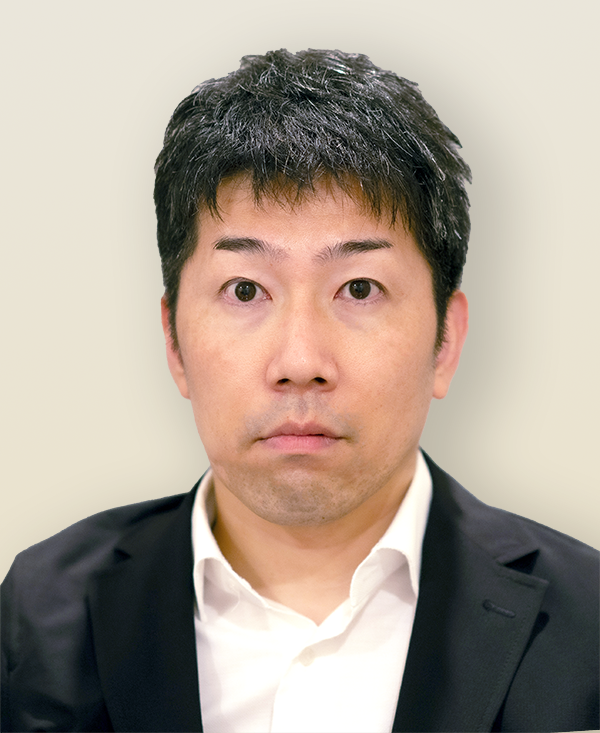
今回は、以前から気になっていたRaspberry Pi AI Camera(以降、AI Camera)を用いて、エッジAIシステムを構築してみました。
AI Cameraとは、IMX500というAI処理を行うイメージセンターが搭載されたカメラです。
Raspberry Pi AI Camera
1.AI cameraのセットアップ
はじめに、Raspberry PiとAI Cameraを接続します。Raspberry Pi 5の場合は、AI Cameraのケーブルを、同梱されている先の細いケーブルに、差し替える必要があります。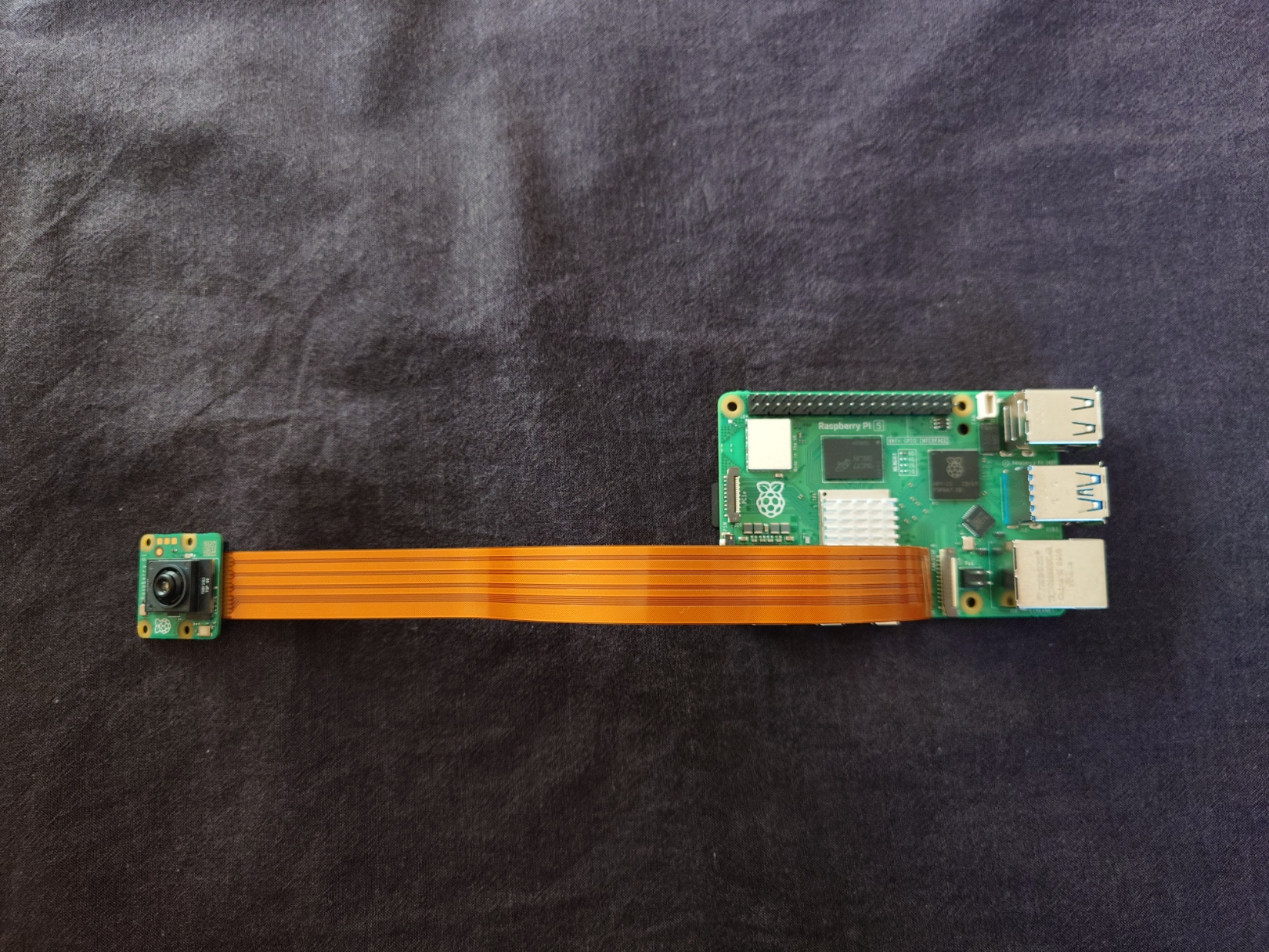
次に、以下に記載されている手順に従ってセットアップを行います。
https://www.raspberrypi.com/documentation/accessories/ai-camera.html
まず、Raspberry Piを最新の状態にします。
$ sudo apt update && sudo apt full-upgrade
IMX500のファームウェアをインストールします。
$ sudo apt install imx500-all
Raspberry Piを再起動します。
$ sudo reboot
再起動したら、AI Camera (IMX500) が認識されていることを確認します。
$ sudo dmesg | grep imx500
[ 0.419205] platform 1f00128000.csi: Fixed dependency cycle(s) with /axi/pcie@120000/rp1/i2c@80000/imx500@1a
[ 3.075421] rp1-cfe 1f00128000.csi: found subdevice /axi/pcie@120000/rp1/i2c@80000/imx500@1a
[ 3.415697] imx500 11-001a: Device found is imx500
[ 3.416234] rp1-cfe 1f00128000.csi: Using sensor imx500 11-001a for capture
AI Cameraに初めから入っている MobileNet-SSDで動作確認をします。
$ rpicam-hello -t 0s --post-process-file /usr/share/rpi-camera-assets/imx500_mobilenet_ssd.json --viewfinder-width 1920 --viewfinder-height 1080 --framerate 30
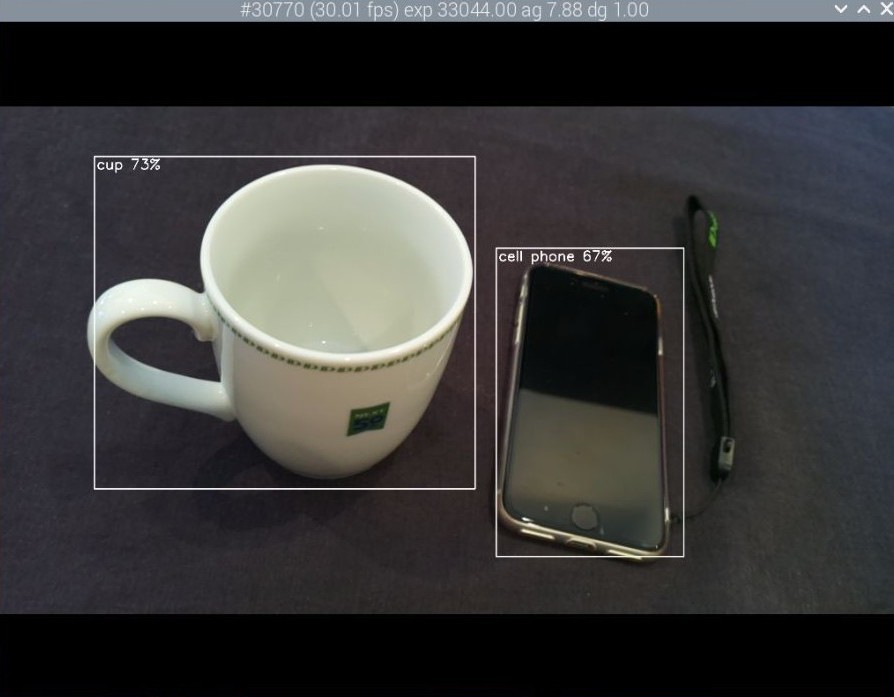
この状態でも物体検知ができていることが確認できますね。
2.独自モデルの構築
実使用においては、独自のモデルを構築してAI Cameraにデプロイすることが多いかと思います。その際は、独自モデルをPC上で作成し、それをAI Cameraで実行できる形式にコンバートして、デプロイします。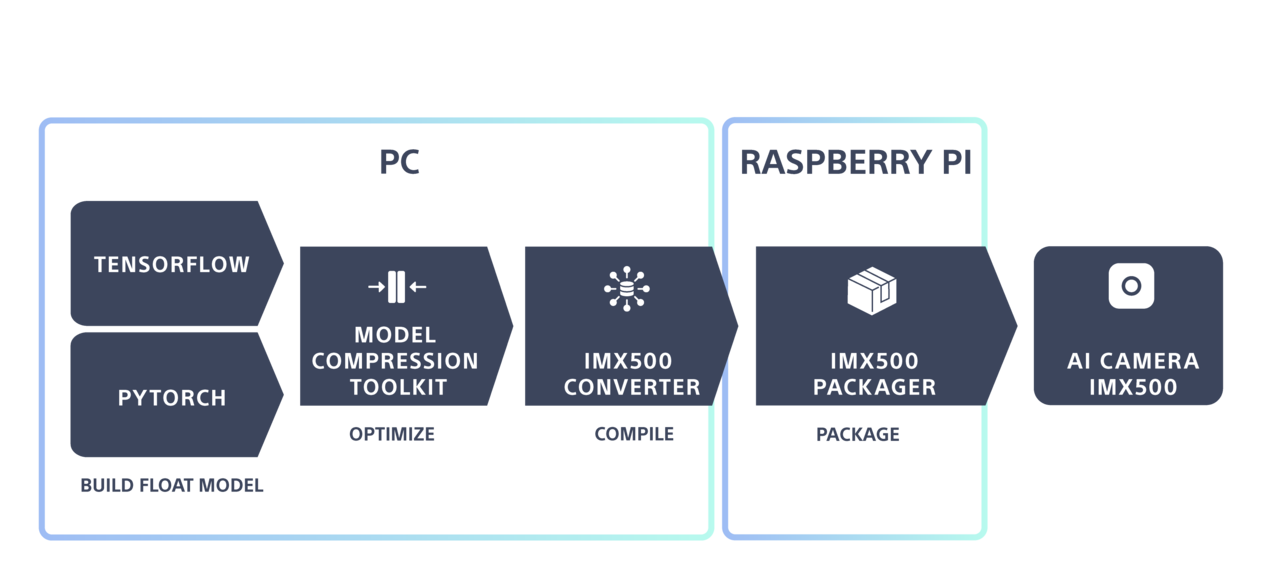
引用: https://developer.aitrios.sony-semicon.com/en/raspberrypi-ai-camera/develop/ai-tutorials/prepare-and-deploy-ai-models-tutorial
今回は独自モデルの例として、学習済みのMobileNetに転移学習を行います。以下のオープンデータセットを追加学習用データとして使用し、犬と猫の画像を分類するモデルを作成します。
The Oxford-IIIT Pet Dataset
■作成するモデルのイメージ
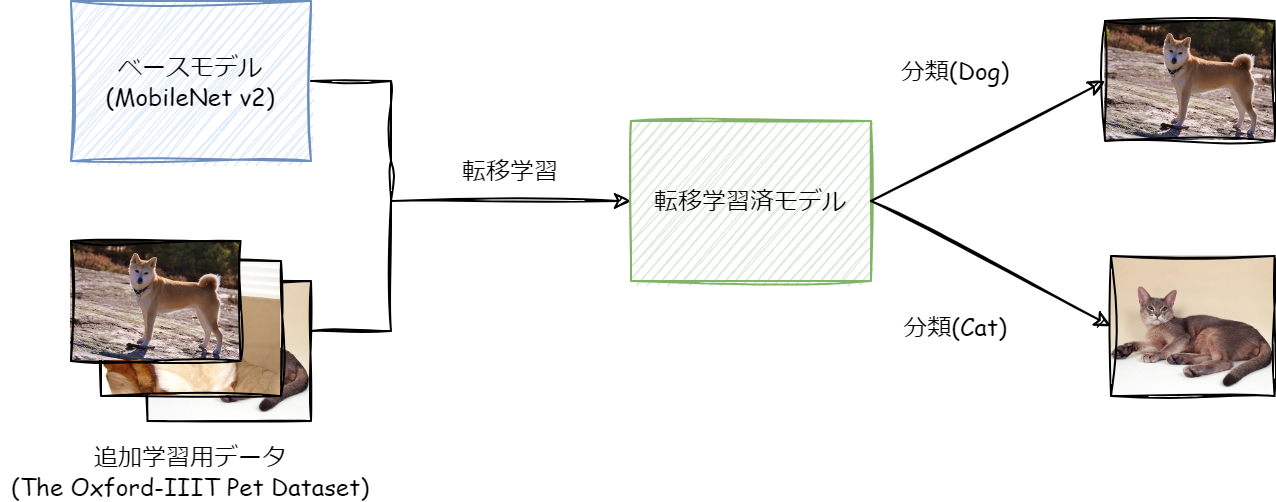
以降は、Pythonのコード例となりますが、Google ColaboratoryなどのNotebook上での逐次実行を想定しています。
データセットの準備
データセットの読み込みは、PyTorchのImageFolderを使用します。入手したデータセットのディレクトリ構成を、ImageFolderで読み込ませるための構成に変更します。■ディレクトリ構成のイメージ
dataset/
├── train/
│ ├── cat/
│ │ ├── Abyssinian_1.jpg
│ │ ├── Bengal_23.jpg
│ │ └── ...
│ └── dog/
│ ├── Beagle_45.jpg
│ ├── Boxer_12.jpg
│ └── ...
└── val/
├── cat/
└── dog/
■コード
import os
import shutil
from sklearn.model_selection import train_test_split
import re
def organize_dataset(source_dir, dest_dir, val_split=0.2):
"""
ファイル名に基づいて画像を猫(cat)と犬(dog)のクラスに分類してデータセットを構築
Parameters:
source_dir: 元の画像があるディレクトリ(すべての画像が1つのフォルダにある)
dest_dir: データセットを作成するディレクトリ
val_split: 検証データの割合
"""
# 猫の品種リスト
cat_breeds = {
"abyssinian", "bengal", "birman", "bombay", "british_shorthair",
"egyptian_mau", "maine_coon", "persian", "ragdoll", "russian_blue",
"siamese", "sphynx"
}
# 画像ファイルの一覧を取得
image_files = [f for f in os.listdir(source_dir)
if f.lower().endswith((".jpg", ".jpeg", ".png", ".bmp"))]
# ファイル名からクラス(猫/犬)を判定
class_images = {"cat": [], "dog": []}
for img in image_files:
# 拡張子を除去
name_without_ext = os.path.splitext(img)[0]
# 末尾の連番を除去(末尾の数字を削除)
breed_name = re.sub(r"\d+$", "", name_without_ext).rstrip("_").rstrip("-").lower()
# 品種名から猫/犬を判定
if any(cat_breed in breed_name for cat_breed in cat_breeds):
class_images["cat"].append(img)
else:
class_images["dog"].append(img)
# クラスごとにディレクトリを作成し、画像を振り分け
for class_name, images in class_images.items():
# trainとvalディレクトリにクラスのサブディレクトリを作成
train_class_dir = os.path.join(dest_dir, "train", class_name)
val_class_dir = os.path.join(dest_dir, "val", class_name)
os.makedirs(train_class_dir, exist_ok=True)
os.makedirs(val_class_dir, exist_ok=True)
# trainとvalに分割
train_images, val_images = train_test_split(
images,
test_size=val_split,
random_state=42
)
# 画像をコピー
for img in train_images:
src = os.path.join(source_dir, img)
dst = os.path.join(train_class_dir, img)
shutil.copy2(src, dst)
for img in val_images:
src = os.path.join(source_dir, img)
dst = os.path.join(val_class_dir, img)
shutil.copy2(src, dst)
print(f"Class {class_name}: {len(train_images)} training images, {len(val_images)} validation images")
■実行例
base_dir = "dataset"
source_dir = "original_images" # すべての画像が入っているディレクトリ
# ディレクトリ構造を作成
os.makedirs(os.path.join(base_dir, "train"), exist_ok=True)
os.makedirs(os.path.join(base_dir, "val"), exist_ok=True)
# 画像を分類して配置
organize_dataset(source_dir, base_dir, val_split=0.2)
独自モデルの準備
ここからは、実際にモデルを実装していきます。ステップごとに関数を作成して、最後にそれを実行します。(1) ライブラリの準備
!pip install -q torch torchvision
!pip install -q onnx==1.16.1
import importlib
if not importlib.util.find_spec("model_compression_toolkit"):
!pip install model_compression_toolkit
import torch
import torch.nn as nn
import torch.optim as optim
from torch.utils.data import DataLoader
from torchvision.models import mobilenet_v2, MobileNet_V2_Weights
from torchvision import transforms, datasets
import numpy as np
import random
from tqdm import tqdm
import model_compression_toolkit as mct
import os
(2)データセットの読み込み
「2.1 データセットの準備」で用意したデータセットを読み込みます。
def create_data_loaders(train_dir, val_dir, batch_size=32):
"""
転移学習用のデータローダーを作成
Parameters:
train_dir (str): 学習データのディレクトリパス
val_dir (str): 検証データのディレクトリパス
batch_size (int): バッチサイズ
"""
# データ拡張と前処理の定義
train_transform = transforms.Compose([
transforms.Resize(256),
transforms.RandomResizedCrop(224),
transforms.RandomHorizontalFlip(),
transforms.ColorJitter(brightness=0.2, contrast=0.2, saturation=0.2),
transforms.ToTensor(),
transforms.Normalize([0.485, 0.456, 0.406], [0.229, 0.224, 0.225])
])
val_transform = transforms.Compose([
transforms.Resize(256),
transforms.CenterCrop(224),
transforms.ToTensor(),
transforms.Normalize([0.485, 0.456, 0.406], [0.229, 0.224, 0.225])
])
# データセットの作成
train_dataset = datasets.ImageFolder(train_dir, transform=train_transform)
val_dataset = datasets.ImageFolder(val_dir, transform=val_transform)
# データローダーの作成
train_loader = DataLoader(
train_dataset,
batch_size=batch_size,
shuffle=True,
num_workers=4,
pin_memory=True
)
val_loader = DataLoader(
val_dataset,
batch_size=batch_size,
shuffle=False,
num_workers=4,
pin_memory=True
)
return train_loader, val_loader, len(train_dataset.classes)
(3)モデルの準備
事前学習済みモデルの読み込みと、転移学習用の修正です。def prepare_model_for_transfer_learning(num_classes, freeze_backbone=True):
"""
転移学習用のMobileNetV2モデルを準備
Parameters:
num_classes (int): 新しいタスクのクラス数
freeze_backbone (bool): バックボーンを凍結するかどうか
"""
# 事前学習済みモデルの読み込み
weights = MobileNet_V2_Weights.IMAGENET1K_V2
model = mobilenet_v2(weights=weights)
if freeze_backbone:
# バックボーン部分のパラメータを凍結
for param in model.parameters():
param.requires_grad = False
# 分類層を新しいタスク用に置き換え
in_features = model.classifier[-1].in_features
model.classifier = nn.Sequential(
nn.Dropout(p=0.2),
nn.Linear(in_features, num_classes)
)
return model
(4) 転移学習の実行
転移学習部分は、PyTorchを用いた標準的な実装です。def train_model(model, train_loader, val_loader, num_epochs=10):
"""
モデルの転移学習を実行
Parameters:
model: 学習するモデル
train_loader: 学習データのDataLoader
val_loader: 検証データのDataLoader
num_epochs (int): エポック数
"""
device = torch.device("cuda:0" if torch.cuda.is_available() else "cpu")
model = model.to(device)
criterion = nn.CrossEntropyLoss()
# 分類層のパラメータのみを最適化
optimizer = optim.Adam(model.classifier.parameters(), lr=0.001)
scheduler = optim.lr_scheduler.ReduceLROnPlateau(
optimizer,
mode="min",
patience=2,
factor=0.1
)
best_val_loss = float("inf")
best_model_state = None
for epoch in range(num_epochs):
# 学習フェーズ
model.train()
train_loss = 0.0
train_correct = 0
train_total = 0
for inputs, labels in tqdm(train_loader, desc=f"Epoch {epoch+1}/{num_epochs}"):
inputs, labels = inputs.to(device), labels.to(device)
optimizer.zero_grad()
outputs = model(inputs)
loss = criterion(outputs, labels)
loss.backward()
optimizer.step()
train_loss += loss.item()
_, predicted = outputs.max(1)
train_total += labels.size(0)
train_correct += predicted.eq(labels).sum().item()
train_loss = train_loss / len(train_loader)
train_acc = 100. * train_correct / train_total
# 評価フェーズ
model.eval()
val_loss = 0.0
val_correct = 0
val_total = 0
with torch.no_grad():
for inputs, labels in val_loader:
inputs, labels = inputs.to(device), labels.to(device)
outputs = model(inputs)
loss = criterion(outputs, labels)
val_loss += loss.item()
_, predicted = outputs.max(1)
val_total += labels.size(0)
val_correct += predicted.eq(labels).sum().item()
val_loss = val_loss / len(val_loader)
val_acc = 100. * val_correct / val_total
# 学習率の調整
scheduler.step(val_loss)
# ベストモデルの保存
if val_loss < best_val_loss:
best_val_loss = val_loss
best_model_state = model.state_dict()
print(f"Epoch {epoch+1}/{num_epochs}:")
print(f"Train Loss: {train_loss:.4f}, Train Acc: {train_acc:.2f}%")
print(f"Val Loss: {val_loss:.4f}, Val Acc: {val_acc:.2f}%")
# ベストモデルの復元
model.load_state_dict(best_model_state)
return model
(5)モデルの評価(転移学習後、モデルの量子化後)
モデルの評価部分です。転移学習後のモデルと、それを量子化した後のモデル双方を評価します。def evaluate_model(model, test_loader):
"""
モデルの評価
Parameters:
model: 評価するモデル
test_loader: 評価データのDataLoader
"""
device = torch.device("cuda:0" if torch.cuda.is_available() else "cpu")
model.to(device)
model.eval()
correct = 0
total = 0
with torch.no_grad():
for data in tqdm(test_loader, desc="Evaluating"):
images, labels = data
images, labels = images.to(device), labels.to(device)
outputs = model(images)
_, predicted = outputs.max(1)
total += labels.size(0)
correct += predicted.eq(labels).sum().item()
accuracy = 100. * correct / total
print(f"Accuracy: {accuracy:.2f}%")
return accuracy
(6)モデルの量子化
モデルを量子化する部分です。def quantize_model(model, train_loader, n_iter=10):
"""
モデルの量子化を実行
Parameters:
model: 量子化するモデル
train_loader: 学習データのDataLoader
n_iter (int): 代表的なデータセットのサイズ
"""
def representative_dataset_gen():
dataloader_iter = iter(train_loader)
for _ in range(n_iter):
yield [next(dataloader_iter)[0]]
# TPCの取得
target_platform_cap = mct.get_target_platform_capabilities("pytorch", "default")
# Post-Training Quantizationの実行
quantized_model, quantization_info = mct.ptq.pytorch_post_training_quantization(
in_module=model,
representative_data_gen=representative_dataset_gen,
target_platform_capabilities=target_platform_cap
)
return quantized_model
(7)メインの実行フロー
ここまでで実装した関数を実行していきます。def main():
# パラメータの設定
train_dir = "<DATASET PATH>/train" # 学習データのパス
val_dir = "<DATASET PATH>/val" # 検証データのパス
batch_size = 32
num_epochs = 10
# 1. データローダーの準備
train_loader, val_loader, num_classes = create_data_loaders(
train_dir,
val_dir,
batch_size
)
# 2. モデルの準備
model = prepare_model_for_transfer_learning(
num_classes,
freeze_backbone=True
)
# 3. 転移学習の実行
print("Starting transfer learning...")
trained_model = train_model(
model,
train_loader,
val_loader,
num_epochs
)
# 4. 転移学習後のモデル評価
print("\nEvaluating transfer learned model:")
float_accuracy = evaluate_model(trained_model, val_loader)
# 5. モデルの量子化
print("\nQuantizing model...")
quantized_model = quantize_model(trained_model, train_loader)
# 6. 量子化モデルの評価
print("\nEvaluating quantized model:")
quantized_accuracy = evaluate_model(quantized_model, val_loader)
# 7. モデルのエクスポート
print("\nExporting model...")
def representative_dataset_gen():
dataloader_iter = iter(train_loader)
for _ in range(10):
yield [next(dataloader_iter)[0]]
mct.exporter.pytorch_export_model(
quantized_model,
save_model_path="quantized_transfer_model.onnx",
repr_dataset=representative_dataset_gen
)
print("\nResults summary:")
print(f"Original model accuracy: {float_accuracy:.2f}%")
print(f"Quantized model accuracy: {quantized_accuracy:.2f}%")
print("Model exported as 'quantized_transfer_model.onnx'")
if __name__ == "__main__":
main()
実行したところ、量子化前後で精度はそれほど変わりませんでした。
Results summary:
Original model accuracy: 98.71%
Quantized model accuracy: 98.51%
Model exported as 'quantized_transfer_model.onnx'
3.独自モデルをRaspberry Piへデプロイ
Raspberry PI へのデプロイは、以下のサイトの手順を参考に実施します。前半はPCで実施し、後半はRaspberry Piで実施します。https://developer.aitrios.sony-semicon.com/en/raspberrypi-ai-camera/develop/ai-tutorials/prepare-and-deploy-ai-models-tutorial?version=2024-11-21&progLang=
コンバート(PC)
サイトの「2.1.3. Compile with IMX500 Converter」を参考に、量子化したモデルを、AI Cameraで実行できる形式にコンバートします。しかし、この手順はWindowsでは実行できないため、WSLなどのLinux環境で実行する必要があります。また、Javaのインストールも必要です。
$ pip install imx500-converter[pt]
$ imxconv-pt -i <MODEL PATH> -o <CONVERTER OUTPUT PATH> --overwrite-output
<CONVERTER OUTPUT PATH>に、packerOut.zipというファイルが生成されるので、これをRaspberry Piに格納します。
さらに、以下のような分類するクラスを記入したファイル(class.txt)を作成して格納します。
cat
dog
パッケージ化(Raspberry Pi)
サイトの「2.2.3. Package model」を参考にパッケージ化を行います(2.2.1. ~ 2.2.2.は、これまでの手順にて実行済みです)。$ sudo apt install python3-opencv python3-munkres
$ imx500-package -i <CONVERTER OUTPUT PATH> -o <RPK OUTPUT PATH>
実行(Raspberry Pi)
サイトの「2.2.4. Visualize model」を参考に実行してみます。$ git clone https://github.com/raspberrypi/picamera2.git
実行に使用するプログラムですが、2クラス分類の場合は、そのまま実行するとエラーとなるため、コピーして編集します。
$ cp picamera2/examples/imx500/imx500_classification_demo.py classification.py
コピーしたclassification.pyを以下のように編集します。
# 30行目:コメントアウトします。
# assert len(LABELS) in [1000, 1001], "Labels file should contain 1000 or 1001 labels."
# 52行目:以下のように変更します。
top_indices = np.argpartition(-np_output, 1)[:2]
プログラムを実行します。
$ python classification.py --model <RPK OUTPUT PATH>/network.rpk --preserve-aspect-ratio --label class.txt --softmax
リアルタイムで画像分類が行われます。dogが0.986(98.6%)となっているので、正しく分類できていることが分かりますね。
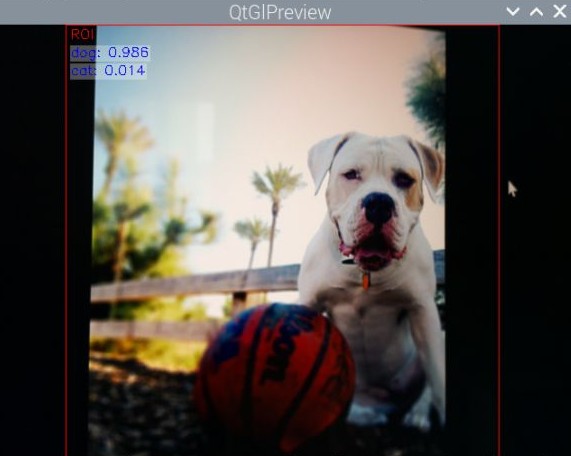
画像分類の結果
まとめ
実機上では、リアルタイム映像を瞬時に推論しており、スピードの速さに驚きました。しかし、ワークロードによっては、実機上の精度が低下することもあり、実務では深い検証が必要になると思います。今回の検証においても、はじめは犬と猫の37種別を分類するモデルで検証しましたが、実機上の精度が上がらず、犬と猫の2クラス分類に変更しました。これらを考慮しても、AIの処理をカメラに任せることができるため、Raspberry Pi本体は、温度センサーなどのセンサー制御や、管理用のWebアプリの実行といった別の処理に専念させることができます。これは大きなメリットだと思います。エッジAIの可能性が多いに広がるのではないかと感じました。
以上、最後までお読みいただきありがとうございました。
当サイトの内容、テキスト、画像等の転載・転記・使用する場合は問い合わせよりご連絡下さい。
エンジニアによるコラムやIDグループからのお知らせなどを
メルマガでお届けしています。